The future of Generative AI is bright — and not as scary as you think
July 10, 2023We’ve all had our minds blown recently by artificial intelligence, and probably more than once. With the release of OpenAI’s ChatGPT to the public in 2022, as Forbes explains it, “a tremor was felt around the world — as the power of AI was democratized to the masses.” After ChatGPT, it was Midjourney. Or Bard. Or GPT-4. And inevitably more to come.
Opinions on the potential of generative AI, since then, have been generally positive — yet tinged with doubt and uncertainty.
- 57% of IT leaders believe generative AI is a ‘game changer.’
- Another third feel it’s overhyped, but still believe generative AI could help their organization better serve customers.
Hopefuls and naysayers alike, we’ve all been using AI in some form for quite some time now. But something has felt different recently. A tide has undeniably turned, and these models are turning out to be so powerful that many people wonder if they’re sentient, and are urging leaders to hit “pause” on their development.
Still, whether or not generative AI is overly hyped (or feared), there is no future in which it can be stuffed back into Pandora’s box. So let’s talk about where we are now, and why you should feel eager about where we’re going — in a world enabled by artificial intelligence.
What’s the difference between generative and predictive AI?
People are talking about generative AI to differentiate it from what we’ve historically had with predictive AI. And while they sometimes get lumped together under a general AI umbrella, it’s important to understand how they differ.
- Predictive AI was generally used to observe data and then make predictions about what it learned (think: which debts will default, where will a hurricane make landfall, which content will most enrage us or enthrall us on social media platforms).
- Generative AI, on the other hand, is tasked with generating new data. Having seen a lot of data, it starts to understand which patterns follow other patterns. “Write a limerick!” we said. And it did. “Give us some fun socks!” we said. And it did.
Today’s Chat GPT-fueled frenzy has put a laser focus on the latter. And it makes sense, because there are millions upon millions of eyes on it. In fact, this January alone, ChatGPT reached 100 million monthly active users, just two months after launch: making it the fastest-growing consumer application in history.
How LLMs make generative AI a lucrative business tool
If you remember one thing about generative AI, it should be this. What makes generative AI such an opportunity-rich business tool? A large part of the story (no pun intended), is about Large Language Models (LLMs).
LLMs are special cases of generative AI that have earned their “large” status due to hundreds of billions of model parameters. LLMs have received widespread attention and been hailed as a game-changer for businesses, having shown impressive results in natural language processing, sentiment analysis, and language translation. But, as with any new technology, there are still limitations and challenges at play.
- LLMs are incredibly powerful, but not perfect — and their effectiveness is dependent on the quality of data they are trained on.
- Essentially, if the data is biased or incomplete, the results generated by AI and LLMs may also be biased or incomplete.
Admittedly, LLMs are still in their early stages of development, but they have the potential to revolutionize the way we interact with computers. And in revolutionizing how we interact with technology, we’re empowered to revolutionize how we connect with each other. For brands, this means technology-enabled teams and better, more meaningfully built customer relationships.
Nonetheless, LLMs being so large has two really important implications for the market, and it’s important to be aware of what we’re up against.
The potential blockers to generative AI and LLM’s future
First and foremost, we should acknowledge that training these models is crazy expensive. We’re talking millions of dollars just in compute costs. And this means people probably won’t be building their own anytime soon.
Additionally, in order to train AI models, businesses need to have access to large amounts of training data. This data can be expensive and time-consuming to collect. This is another reason people probably won’t be building their own. And within many organizations, there are not enough people with the skills to develop and implement AI and LLMs. This is a major bottleneck preventing businesses from adopting these technologies, especially since the data teams in charge of training these models often end up spending most of their time finding, preparing, and cleaning data instead.
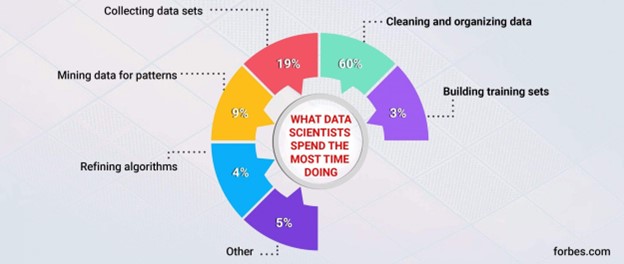
Nonetheless, we know that these models have seen a lot of data, terabytes of data, in fact. AI can write new limericks because it has seen tons of limericks before. They utilize a novel, underlying set of foundation models that can be adapted to the new ways in which you want to use it. This is true even if those ways weren’t known when the model was trained. This process is known as fine-tuning, and it’s the reason you’ve seen LLMs being used on such a wide variety of tasks.
Heading toward an AI-assisted reality
With a hammer as sexy as LLMs, people are going to be finding lots of different kinds of nails. Software engineers and data scientists will be looking to transform problems into a form that generative AI can solve in a process known as prompt engineering. Everyone else will be looking for ways to push the boundaries of what we’re using these models to solve.
Generative AI should be making you re-evaluate the way that you work. Imagine you had an AI-assistant that automated everything that you type. What decisions would you still be making? How many smarter, faster decisions could you make?
The reality for the foreseeable future is that most of the usage of generative AI will be used to assist us, not replace us. AI won’t replace lawyers. The lawyers who use AI will replace the lawyers who don’t. But the story of generative AI is much more than just a will-they-won’t-they about an AI workforce.
AI + CDP = More than a jumble of letters
One area where AI and LLMs are having a major impact is in the field of customer data platforms (CDPs).
CDPs collect and store customer data from a variety of sources, using insights from that data to create a single view of the customer, and democratize data access to improve marketing, sales, and customer service.
AI and LLMs hold immense potential for CDPs. They can be used to analyze customer behavior, identify patterns, and generate personalized recommendations. For example, an AI-powered CDP can analyze a customer’s purchase history and recommend products they are likely to be interested in based on their previous purchases. Additionally, LLMs can help businesses analyze customer feedback and sentiment, enabling them to better understand their customers’ needs and improve their products and services.
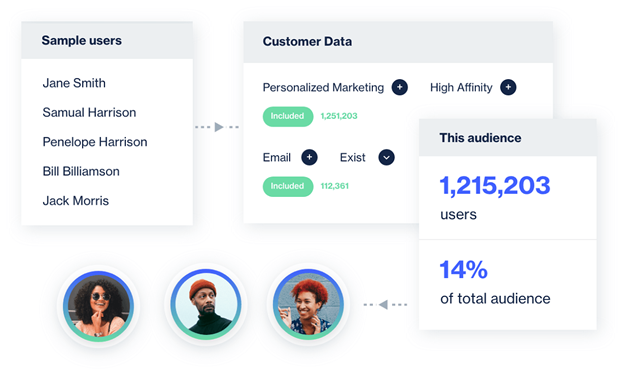
AI and LLMs can also be used to power a variety of features in CDPs. For example, AI can be used to identify patterns in customer data, which can then be used to segment customers into different groups. LLMs can be used to generate personalized content for each customer segment.
The future of AI and LLMs in CDPs is very bright. As these technologies continue to develop, they will become even more powerful and sophisticated. This will allow CDPs to provide even more value to businesses by helping them to better understand their customers and deliver more personalized experiences.
How Lytics CDP fits into the generative AI-led future
With a customer data platform (CDP) like Lytics, you are always on the hook for understanding why you’re bringing data together, and what it is that you want to do with the result. The rest is just implementation detail — mapping, routing, identity rules, audience rules and segmentation logic.
You’ll see an increasing amount of the implementation get automated away by new AI technologies. At Lytics, we’ve started with two important advances. For data stakeholders, we’ve launched Schema Copilot, which uses LLMs to generate schema mappings and identity resolution suggestions to provide better consistency in your data and accelerate your implementation. For business stakeholders, we’ve launched the Audience Generator, which helps you translate your business requirements from natural language into query languages like SQL. Both products turn generative AI tools into toolkits that make using your data ever more accessible.
It’ll never be a question of whether data engineers or marketers get replaced by AI tools. It will always be a question of which tasks the AI will own, and what kind of tasks that will free you up to do. As humans, we bring our passions, our objectives, our guidance. The machine will bring a growing share of the rest.
This article was originally published on Lytics website. Click here to see the original blog post.